The Threat of Artificial Intelligence in a Post-Truth Political Landscape
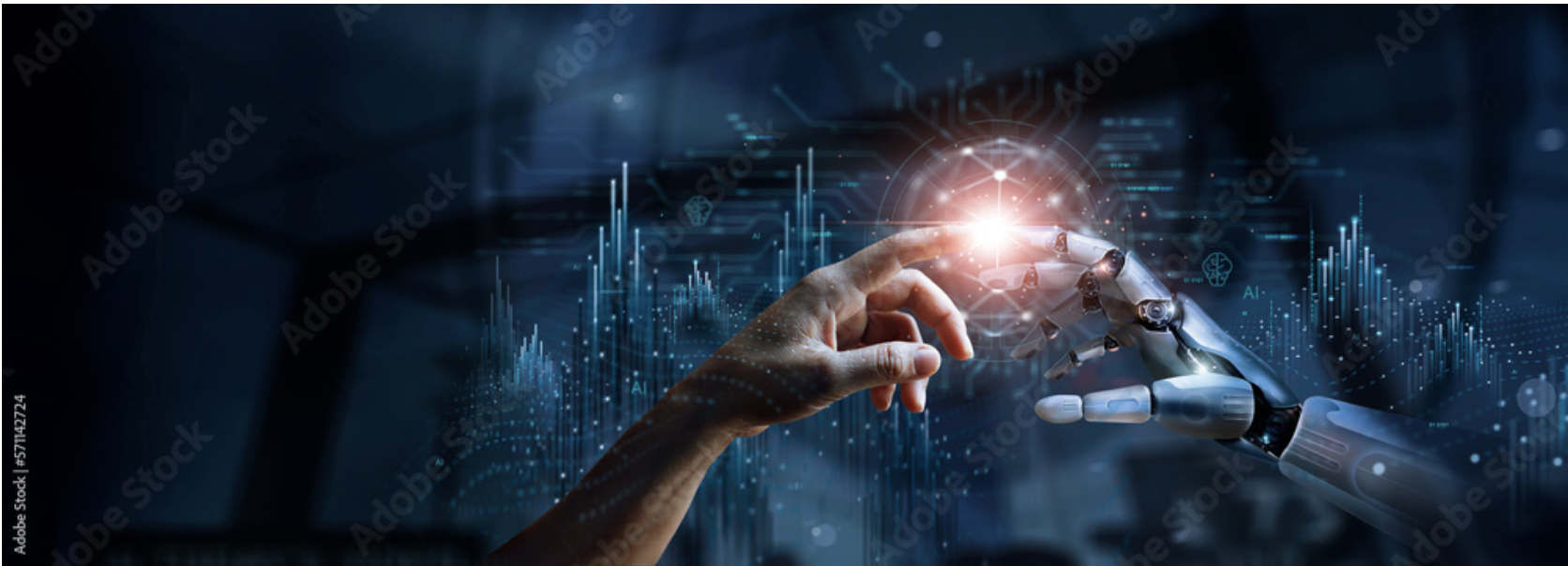
Artificial intelligence has captured the collective imagination. These technologies have advanced rapidly, and their development is unlike anything we have seen before. We have found, thus far, that artificial intelligence systems that run using machine learning excel at imitating human language, art, and other types of data. As a result, these systems are able to generate large quantities of media that closely mimic media created by humans. The scalability of this technology raises questions about its potential usage by negligent or malignant actors to create post-trust media, which is content that has no relation to the truth and is often used to disinform. While the existing post-truth nature of national and local political media environments poses a distinct threat to political discourse and institutions, the advancement of artificial intelligence-based language models exacerbates this threat, making it more imminent and existential than previously assumed. Therefore, developments in artificial intelligence necessitate a more deliberate and immediate policy response.
-
The Post-Truth Political Landscape (And Why It’s Bad)
“Post-truth” is a term used to describe an environment where news stories’ probative value is overshadowed by a need to appeal to the audiences’ emotions or personal learnings [2]. The surplus of media in the modern news ecosystem allows post-truth environments to flourish; it enables the quantity of published stories and their respective injection of pathos to outweigh the need for quality reporting.
Notably, there is a subtle difference between news that is fake and news that is “post-truth”. Although they are alike in their falseness, fake news implies an intent to deceive the reader and a knowledge that the information being disseminated is false. By contrast, post-truth refers to media that bears no connection to the truth. The best way to conceptualize this dichotomy is from the perspective of the author: while the author of fake news knows the truth and intends to distract or deceive from it, the post-truth author either does not know or does not care about the truth and merely intends to create watchable, readable, or listenable content. While fake news is arguably more sinister on its own, the current economic structure of the internet – that pays content creators for clicks and shares through ad revenue – incentivizes bold, emotional, and interesting content [3]. Consequently, creators face pressure to generate stories that are as outrage-worthy or arousing as possible, leading some to conceive entirely new narratives, known as post-trust news.
The implications of a media environment in which viewers can no longer trust the media they consume for accuracy, or one in which they can no longer discern factual from nonfactual stories, are drastic for our political system. It has led to segregated media environments that present wildly different perspectives on the same stories or different stories altogether, ultimately exacerbating political polarization. As media sources become increasingly polarized, audiences tend to seek out news stories that reaffirm their preexisting beliefs, thus perpetuating the segregation of media outlets by political views. For instance, 93% of adult Republicans name Fox News as their primary source of political news compared to just 6% of Democrats, and the numbers are even more skewed for Democratic viewership with MSNBC [4]. This partisan viewership incentivizes media outlets to produce partisan content, and while they may still be legally accountable for misinformation, they have room to frame stories in a way that will maximize views from their respective partisan base. What this environment creates is two entirely separate media spheres and, as a result, a divided electorate operating not just on separate sets of opinions but separate sets of factual information as well.
[5]
The most novel development in the post-truth media sphere comes from the advent of social media. In early studies on the political effects of social media, researchers determined that more than half of online political discussions exposed viewers to agreeing viewpoints, while only around 10% exposed users to disagreeing viewpoints [6]. As these platforms have developed, social media algorithms that optimize viewership on their platforms have contributed to polarization. Researchers of the American Economic Association found that abstaining from social media for a month resulted in individuals’ political views becoming significantly less polarized than those who continued to use social media [7]. These algorithms incentivize content that uses moral and emotional language, which, while not mutually exclusive with factual and informative language, leads to less value on true knowledge and increased value on political conformity [8]. As such, the software technology behind media platforms, while not solely to blame, bears responsibility in shaping the present post-truth political media dynamic.
-
Current Capabilities of Artificial Intelligence and the Threat They Pose
The recent widespread release of ChatGPT, a language-modeled chatbot created by OpenAI and modeled after Generative Pretrained Transformer-3, has brought artificial intelligence, its capabilities, and its shortcomings to the forefront of tech-related conversations. The technology is created using language processing and statistical modeling, meaning that OpenAI trained the algorithm on over 175 billion pieces of real data from the English language and then rated the system's responses to prompts to train the system [9]. This causes the system to develop “emergent capabilities” or capabilities that aren’t otherwise coded into the model [10]. Its responses are hyper-realistic, mimicking human language patterns across a variety of literary and linguistic styles. OpenAI’s founder, Sam Altman, indicates that he wishes to create what is known as “artificial general intelligence”, or a computing system that is fully capable of reasoning and thinking the way a human can [11]. However, because ChatGPT is currently limited to responding to text and media-based prompts, it falls into the category of “artificial narrow intelligence”.
[12]
These language processing models create a mimicry of human writing, but they do not recreate the reasoning behind it. AI scholar Gary Marcus details this process with examples such as a user instructing the system to detail how crushed porcelain improves the quality of breast milk, and the system replies: “‘porcelain can help to balance the nutritional content of the milk, providing the infant with the nutrients they need to help grow and develop” [13]. Current developments in artificial intelligence universally rely on large language models, and while they vary in what they are trained to do, the computing method remains mostly the same across different models. The mainstream philosophy on these language processing models is that greater parameter inputs yield greater accuracy and will eventually yield a general intelligence. However, while an increase in the number of parameters increases the likeness of the system’s responses to the input parameters, that increase does not necessarily equate to reasoning. In fact, researchers at UCLA tested a large language model on a scale of pure reasoning they developed called SimpleLogic and found that “though [it] can achieve near-perfect performance on a data distribution that covers the whole space, it always fails to generalize to other distributions that are even just slightly different” [14]. These models rely on statistical similarities to it and, crucially, do not emulate reasoning itself.
Reasoning is an essential step to discerning truth from disinformation. ChatGPT’s and other AI’s current language processing model relies on past data to create future information, but, especially in an ever-changing news-media environment, the past does not always give an accurate indication of the future. Further, because OpenAI trained their system on such a large data set and because they pulled that data set from the internet, there is no guarantee that the system does not develop biases. In fact, bias in AI models caused by programming from biased data sets is incredibly common. When researchers train AI agents on data sets that “capture the results of a history [of] social injustice,” it “exacerbates existing discriminatory practices” [15]. The internet already contains significant bias and misinformation, and because artificial intelligence firms are not legally required to disclose their data set, government agencies, and media outlets have no way to discern whether their model or any future model is trained on biased data.
Large language models, especially if developed in private, offer users the ability to create any media they choose on an unprecedented scale. As Ezra Klein describes it, it “drive[s] the cost of bullshit to zero” [16]. Klein derives his definition of “bullshit” from philosopher Harry Frankfurt, who defines it as information that has “no real relationship to the truth” [17]. The scale of this media creation means that while an author at a media outlet could initially create one or two stories per hour, they could create exponentially more assisted by a large language model. This quantity will come at the expense of fact-checking and quality journalism, and because the large language model lacks the ability to reason, there is no effective failsafe mechanism to prevent the spread of misinformation on an unprecedented scale. Therein lies the phenomenon of how large language models, by their inherent nature, are built to enable post-truth discourse. The phenomenon we are experiencing is not that these models have become omniscient; it is that we believe that they are, and it is that belief and trust that enables this technology to pose an imminent threat to our media and political institutions.
-
What Can We Do About It
Large language models already exist, so effectively regulating prior developments in this technology will be difficult. However, due to the current limitations of artificial intelligence and uncertainty about whether planned improvements will benefit the technology, government actions should reflect caution about the potential implications of this technology to political media. They should first work to create liability for AI researchers and software companies for the effects of their technology to both hold them legally accountable and to provide them with a monetary incentive to encourage caution when building these systems. Philosopher Helen Nissenbaum details that there are inherent limitations to liability in computing, including that the tech industry “‘demands maximal ownership’” over their products while insisting that they are not liable for the effects of their media platforms [18]. This lack of legal liability for disinformation and libel means that tech companies are not required to consider the implications of the distribution of their technology. Until the government strictly formalizes this liability, companies will not have an incentive to self-regulate.
The European Union has already taken the first step toward regulating artificial intelligence, and the US should mirror its actions to present a unified front against damaging uses of AI. Their recent legislation package creates a regulatory process for artificial intelligence models, including examining their data sets for bias, before they hit the market [19]. Further, it requires that these systems disclose their algorithm and methodology of how they reached a certain output when given the user’s input [20]. This negates the “black box” effect of regulators not knowing how these systems work, limiting their ability to create legal liability for tech companies. Finally, it enshrines this liability by ensuring that victims are “compensated for damages caused by [...] digital products and AI systems” [21].
Artificial intelligence technologies develop at an increasingly rapid pace, and responses to the threat they pose must be dynamic and evolving. In an environment where truth is increasingly disregarded and political media and discourse are under attack, artificial intelligence represents an existential threat to the legitimacy of democratic political institutions. It is crucial to act carefully to utilize the revolutionary advancements in artificial intelligence without leaving our political institutions and discourse vulnerable to undue influence.
Sources
1. Credit: ipopba - stock.adobe.com
2. Populism Studies. “Post-Truth Politics,” n.d. https://www.populismstudies.org/Vocabulary/post-truth-politics/.
3. New York University. “Messages with Moral-Emotional Words Are More Likely to Go Viral on Social Media.” June 26, 2017. https://www.nyu.edu/about/news-publications/news/2017/june/messages-with-moral-emotional-words-are-more-likely-to-go-viral-.html.
4. Grieco, Elizabeth. “Americans’ Main Sources for Political News Vary by Party and Age.” Pew Research, April 1, 2020. https://www.pewresearch.org/fact-tank/2020/04/01/americans-main-sources-for-political-news-vary-by-party-and-age/.
5. Grieco, Elizabeth. “Americans’ Main Sources for Political News Vary by Party and Age.” Pew Research, April 1, 2020. https://www.pewresearch.org/fact-tank/2020/04/01/americans-main-sources-for-political-news-vary-by-party-and-age/.
6. Tucker, Joshua, Andrew Guess, Pablo Barberá, Cristian Vaccari, Alexandra Siegel, Sergey Sanovich, Denis Stukal, and Brendan Nyhan. “Social Media, Political Polarization, and Political Disinformation: A Review of the Scientific Literature.” Hewlett Foundation, n.d. https://www.forumdisuguaglianzediversita.org/wp-content/uploads/2021/05/Social-Media-Political-Polarization-and-Political-Disinformation-Literature-Review.x28591.pdf.
7. Allcott, Hunt, Luca Braghieri, Sarah Eichmeyer, and Matthew Gentzkow. “The Welfare Effects of Social Media.” American Economic Review 110, no. 3 (March 1, 2020): 629–76. https://doi.org/10.1257/aer.20190658.
8. New York University. “Messages with Moral-Emotional Words Are More Likely to Go Viral on Social Media.” June 26, 2017. https://www.nyu.edu/about/news-publications/news/2017/june/messages-with-moral-emotional-words-are-more-likely-to-go-viral-.html.
9. Hughes, Alex. “ChatGPT: Everything You Need to Know about OpenAI’s GPT-3 Tool.” British Broadcasting Company, February 2, 2023, sec. Future Technology. https://www.sciencefocus.com/future-technology/gpt-3/.
10. Hughes, Alex. “ChatGPT: Everything You Need to Know about OpenAI’s GPT-3 Tool.” British Broadcasting Company, February 2, 2023, sec. Future Technology. https://www.sciencefocus.com/future-technology/gpt-3/.
11. Roose, Kevin. “How ChatGPT Kicked Off an A.I. Arms Race.” New York Times, February 3, 2023, sec. The Shift. https://www.nytimes.com/2023/02/03/technology/chatgpt-openai-artificial-intelligence.html?smid=nytcore-ios-share&referringSource=articleShare.
12. Credit: Atria Innovation - https://www.atriainnovation.com/en/how-does-chat-gpt-work/
13. Marcus, Gary. “AI Platforms like ChatGPT Are Easy to Use but Also Potentially Dangerous.” Scientific American, December 19, 2022. https://www.scientificamerican.com/article/ai-platforms-like-chatgpt-are-easy-to-use-but-also-potentially-dangerous/.
14. Zhang, Honghua, Liunian Harold Li, Tao Meng, Kai-Wei Chang, and Guy Van den Broeck. “On the Paradox of Learning to Reason from Data,” May 24, 2022. http://arxiv.org/abs/2205.11502.
15. Leavy, Susan, Barry O’Sullivan, and Eugenia Siapera. “Data, Power and Bias in Artificial Intelligence,” July 28, 2020. http://arxiv.org/abs/2008.07341
16. Klein, Ezra. “A Skeptical Take on the A.I. Revolution.” The Ezra Klein Show, n.d. https://www.nytimes.com/2023/01/06/podcasts/transcript-ezra-klein-interviews-gary-marcus.html.
17. Klein, Ezra. “A Skeptical Take on the A.I. Revolution.” The Ezra Klein Show, n.d. https://www.nytimes.com/2023/01/06/podcasts/transcript-ezra-klein-interviews-gary-marcus.html.
18. Yamazaki, Tatsuya, Kiyoshi Murata, Yohko Orito, and Kazuyuki Shimizu. “Post-Truth Society: The AI-Driven Society Where No One Is Responsible.” ETHICOMP, July 2020. https://www.researchgate.net/publication/343099318_Post-Truth_Society_The_AI-driven_Society_Where_No_One_Is_Responsible.
19. European Commission. “A European Approach to Artificial Intelligence | Shaping Europe’s Digital Future,” February 6, 2023. https://digital-strategy.ec.europa.eu/en/policies/european-approach-artificial-intelligence.
20. European Commission. “A European Approach to Artificial Intelligence | Shaping Europe’s Digital Future,” February 6, 2023. https://digital-strategy.ec.europa.eu/en/policies/european-approach-artificial-intelligence.
21. European Commission. “A European Approach to Artificial Intelligence | Shaping Europe’s Digital Future,” February 6, 2023. https://digital-strategy.ec.europa.eu/en/policies/european-approach-artificial-intelligence.