America’s Healthcare System is Broken—Is AI the Path Forward?
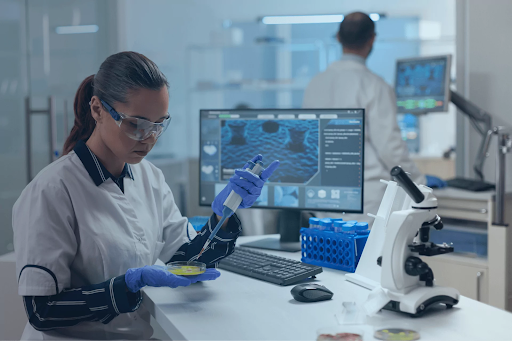
America’s flailing healthcare system has been the target of political discourse and strategy spanning administrative, partisan, and ideological arenas. Recent conversations have centered around policy—specifically, legislation dealing with the scope of federal health insurance such as Medicaid as well as the expansion of privatized healthcare. However, one aspect of healthcare that has been largely overlooked is already beginning to transform the healthcare system. Artificial intelligence (AI) holds the potential to exponentially reduce hospital administration costs, increase access to healthcare, and tackle the most exigent issues that plague the healthcare system today. With deregulation defining the political status quo, the environment is situated to allow AI to expand. The use of AI has already become pervasive within the industry, and its widespread integration seems both inevitable and imminent. However, while AI has the capability to be a tremendous positive tool for the healthcare industry, its rapid expansion must be cautious, using unbiased, accurate data and secure systems integrated with human oversight to avoid the replication of historical prejudice.
The cost of healthcare spending in the United States reached 4.5 trillion dollars in 2022 [1]. In fact, one out of every five dollars in American GDP is spent on healthcare [2]. A fourth of adults skipped health care they needed in the past year due to medical costs and a third skipped a prescription for the same reason. These numbers are more alarming in the uninsured population, with 60 percent skipping care. Further, 40 percent of all adults have medical or dental debt, and 75 percent are concerned with unexpected medical bills [3]. There is both a cost and coverage problem in American healthcare, but also an equity problem. The country is experiencing physician shortages, especially in rural areas where many physicians are overtasked with administrative work due to a lack of support staff [4]. However, this is not merely a rural hospital issue, but rather a systemic problem that drives up healthcare costs writ large.
Within these circumstances, AI derives much of its value, streamlining the most laborious parts of healthcare administration and garnering 1 trillion dollars in improvement potential. Specifically, generative AI can automate patient visit summaries, customize provider-to-individual communication, summarize claims, and perform analytics. It can also make insurance more accessible, summarizing denial letters, taking care of health insurance prior authorization, and creating custom benefit summaries [5]. This places valuable time back into clinicians’ hands, allowing them to focus more on patient care and higher-level tasks. Not only can it improve efficiency, but AI can also improve care by making treatment plans more individualized by analyzing patient genetics and history. AI can identify patterns in data, allowing for more accurate diagnoses and early detection that could improve outcomes for diseases like cancer. It can also synthesize unstructured data like patient records and clinical notes, enabling higher-quality clinical assessments. AI benefits drug development as well, using analytics to identify potential drug candidates, making research quicker and more cost-efficient [6]. Lastly, it holds the potential to foster more equitable healthcare, increasing access to healthcare in underserved communities by helping to identify where resources are needed and freeing up time for overtasked rural physicians while improving quality of care.
Of course, rapidly integrating technology as novel as AI rarely comes without its drawbacks. One such problem that has arisen is reminiscent of issues that plagued many past algorithms trained on real data—the replication of systemic biases or inequalities. A popular but unnamed algorithm that serves millions of Americans lets white people cut in line for programs for patients with chronic conditions while underestimating the health needs of hospitals’ black patients. This led to concrete disparities, as the proportion of black patients who received extra help was reduced by more than half. According to the algorithm’s creators, the software was designed to predict future health costs but was based on data that “predicted” those with lower incomes to be more costly, as they are less likely to have health insurance or the job security necessary to easily attend medical appointments. This is not to claim that the algorithm is inherently racist, but that it replicates inequities that communities of color often face in American society [7]. In addition, the Trump administration has recently rescinded Biden’s executive order on the “safe, secure, and trustworthy development and use of artificial intelligence.” This decision removed crucial guardrails such as safety testing, algorithm bias, and data security, which makes it even more imperative that the healthcare system does not rush to widely institute AI [8]. If AI is not trained with tools that mirror the diversity of American society, it may provide responses that fail to identify symptoms or recommend treatments that harm some individuals [9]. A study by the University of Minnesota analyzed more than 2,000 hospitals across the country and found that disparities in AI development and integration are already present. Only 44 percent of hospitals evaluate their AI models for bias, meaning hospitals that are less resourced are tasked with the decision of either using AI without the necessary oversight or not using it at all [10].
Thus, while AI can be greatly beneficial in reducing administrative costs, it must be integrated with caution. AI tools must be thoroughly tested, assessing performance across different groups and correcting mistakes or biases that may be found, whether through partnering with organizations that can assist with scientific and social testing or setting industry standards for AI [11]. An example of an innovation that may help with these issues is retrieval augmented generation (RAG) AI. It uses models that are trained on newer information, learning from the latest research, clinical guidelines, and patient data. It can use an organization’s specific database to generate results, allowing it to answer questions more specific to its users. While traditional large language models must be retrained to update their knowledge bases, these RAGs are constantly updating and improving in real time [12]. This allows for a more representative algorithm that may diminish biases, while still maintaining the benefits of generative AI, such as navigating unstructured data. Perhaps more important than any innovation or new technology, however, is the persistent maintenance of human-in-the-loop structures. Having a clinician, physician, or other health professional reviewing responses prevents inaccuracies and biases, and maintains HIPAA by ensuring that sensitive information isn’t being leaked [13]. The overreliance on AI without human supervision risks adverse effects, such as a rise in insurance denials, which are slowly becoming more apparent. The largest U.S. insurance companies, such as UnitedHealth, Humana, and Cigna, are currently facing lawsuits for using AI algorithms that have denied lifesaving care. These algorithms were allegedly averaging 1.2 seconds per physician-reviewed claim, resulting in a 90 percent error rate where the vast majority of decisions were reversed upon appeal. The appeals alone led to 7.2 billion dollars in administrative costs, demonstrating how a lack of human oversight may reverse the benefits of using AI in healthcare [14].
AI has the potential to revolutionize healthcare, making it more efficient, accessible, and cost-effective for all. However, its rapid integration must be met with careful oversight. With the current presidential administration removing guardrails, it is imperative that humans remain in the equation, maintaining careful surveillance of AI’s evolution in healthcare. Without these safeguards, AI risks replicating the very problems it seeks to solve, from fostering discrimination to driving up administrative costs. If implemented responsibly, AI could be a powerful tool in reshaping American healthcare for the better. A regulatory framework that focuses on rigorous testing and proactive developmental revision, such as the adoption of RAGs, can and should be implemented, but the healthcare industry must be careful in preventing the replicating of historical trends of inequity.
Sources
[1] Roberts, Brooklyn. “Artificial Intelligence Is Transforming America’s Healthcare - American Legislative Exchange Council.” American Legislative Exchange Council, January 17th, 2024. https://alec.org/article/artificial-intelligence-is-transforming-americas-healthcare/.
[2] Schreiber, Melody. “New AI Tool Counters Health Insurance Denials Decided by Automated Algorithms.” The Guardian, January 25th, 2025. https://www.theguardian.com/us-news/2025/jan/25/health-insurers-ai.
[3] Lopes, Lunna, Alex Montero, Marley Presiado, and Liz Hamel. “Americans’ Challenges With Health Care Costs | KFF.” KFF, May 7th, 2024. https://www.kff.org/health-costs/issue-brief/americans-challenges-with-health-care-costs/.
[4] Roberts, “Artificial Intelligence is Transforming America’s Healthcare.”
[5] Bhasker, Shashank, Damien Bruce, Jessica Lamb, and George Stein. “Tackling Healthcare’s Biggest Burdens With Generative AI.” McKinsey & Company, July 10th, 2023. https://www.mckinsey.com/industries/healthcare/our-insights/tackling-healthcares-biggest-burdens-with-generative-ai.
[6] Roberts, “Artificial Intelligence is Transforming America’s Healthcare.”
[7] Simonite, Tom. “A Health Care Algorithm Offered Less Care to Black Patients.” WIRED, October 24th, 2019. https://wired.com/story/how-algorithm-favored-whites-over-blacks-health-care/.
[8] Muoio, Dave, Noah Tong, Emma Beavins, and Anastassia Gliadkovskaya. “What Trump’s busy first week means for healthcare: AI policy shifts, HHS comms blackout, patient protections and more.” FIERCE Healthcare. January 24th, 2025. https://www.fiercehealthcare.com/regulatory/what-trumps-first-day-orders-mean-healthcare-ditched-drug-models-pauses-rules-and-hiring.
[9] Kasman, Matt, and Ross A. Hammond. “Why And How Should We Regulate the Use of AI in Health Care?” Brookings, September 5th, 2024. https://www.brookings.edu/articles/why-and-how-should-we-regulate-the-use-of-ai-in-health-care/.
[10] Eddy, Nathan. “AI Bias Evaluation Efforts Are Uneven Across U.S. Hospitals.” Healthcare IT News, January 24th, 2025. https://www.healthcareitnews.com/news/ai-bias-evaluation-efforts-are-uneven-across-us-hospitals.
[11] Kasman, “Why and How Should We Regulate the Use of AI in Health Care?”
[12] Eastwood, Brian. “How Does Retrieval-Augmented Generation (RAG) Support Healthcare AI Initiatives?” Technology Solutions That Drive Healthcare, January 27th, 2025. https://healthtechmagazine.net/article/2025/01/retrieval-augmented-generation-support-healthcare-ai-perfcon.
[13] Bhasker, “Tackling Healthcare’s Biggest Burdens with Generative AI.”
[14] Schreiber, “New AI Tool Counters Health Insurance Denials Decided by Automated Algorithms.”